The Rise of Edge AI: Processing Power at the Edge of Networks
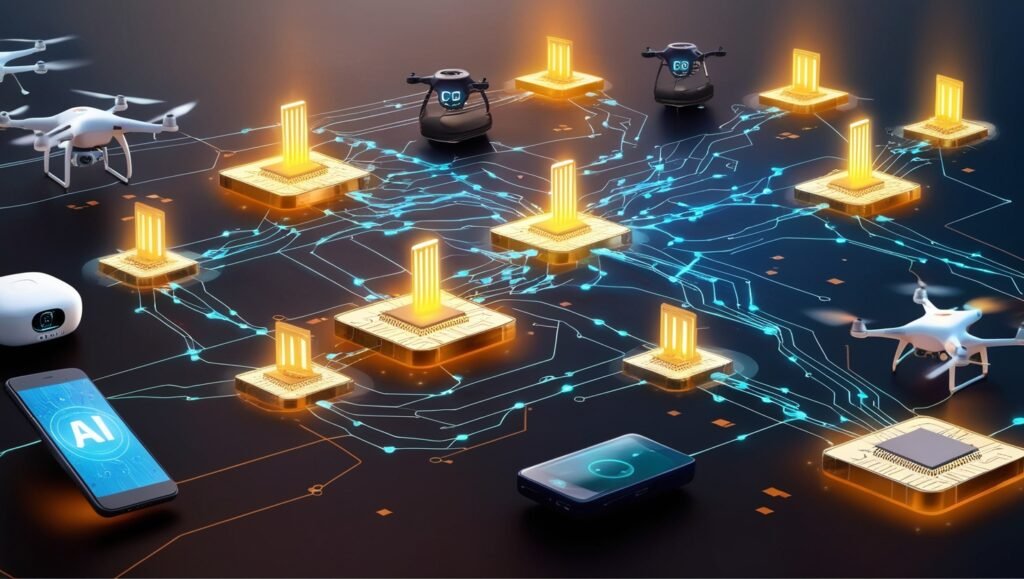
The world of artificial intelligence (AI) is evolving rapidly, and Edge AI has emerged as a transformative technology. Unlike traditional AI systems that rely on centralized cloud computing, Edge AI processes data locally, at the source of data generation. This paradigm shift is enabling faster decision-making, enhancing privacy, and opening new frontiers for innovation across industries. But what exactly is Edge AI, and how did it come about? Let’s explore.
Table of Contents
ToggleWhat is Edge AI?
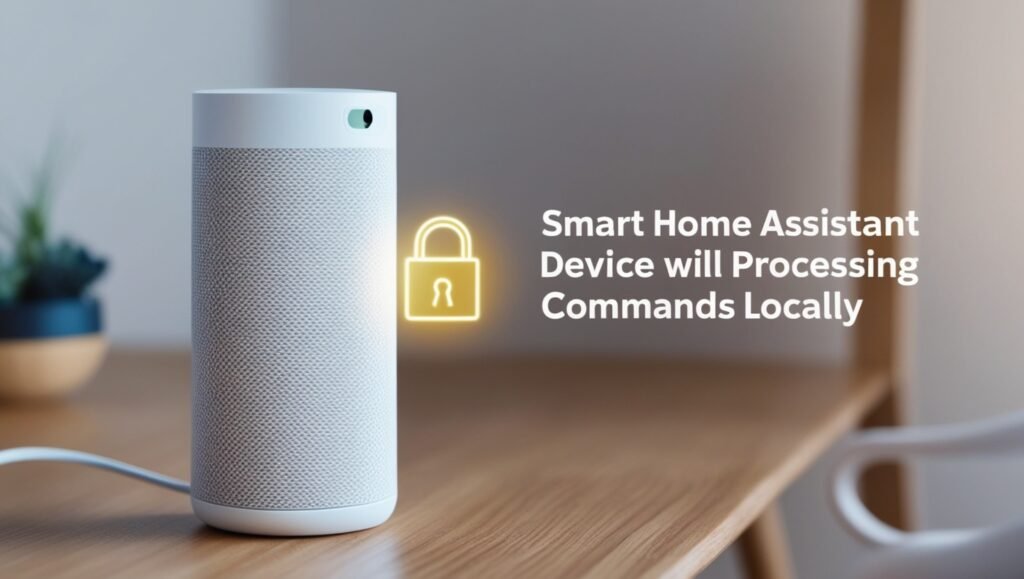
Edge AI refers to deploying artificial intelligence algorithms directly on edge devices, such as smartphones, IoT sensors, drones, and industrial robots. These devices are equipped with the capability to process and analyze data locally without needing to send it to centralized servers. This localized processing reduces latency, improves efficiency, and addresses privacy concerns by keeping sensitive data close to its source.
Example, virtual assistants like Google Assistant and Amazon Alexa increasingly use on-device AI to process voice commands in real-time, offering quicker responses while maintaining user privacy.
The First Steps in Edge AI Development
Edge AI’s origins lie in the development of specialized hardware designed for local data processing. The development of Edge AI started in the mid-2010s with the introduction of specialized hardware designed for local data processing. In 2014, NVIDIA launched its Jetson Series, compact and powerful platforms capable of handling complex AI tasks on edge devices. In 2017, Intel introduced the Movidius Neural Compute Stick, a plug-and-play device that made running deep learning models on edge devices more accessible for developers and researchers. These early innovations played a crucial role in integrating AI into everyday tools like smartphones, drones, and IoT devices, laying the foundation for the rapid advancements we see today.
Applications of Edge AI
Edge AI is revolutionizing industries by enabling real-time data processing for applications like autonomous vehicle navigation, predictive maintenance in manufacturing, personalized healthcare through wearables, energy management in smart grids, and precision farming techniques.
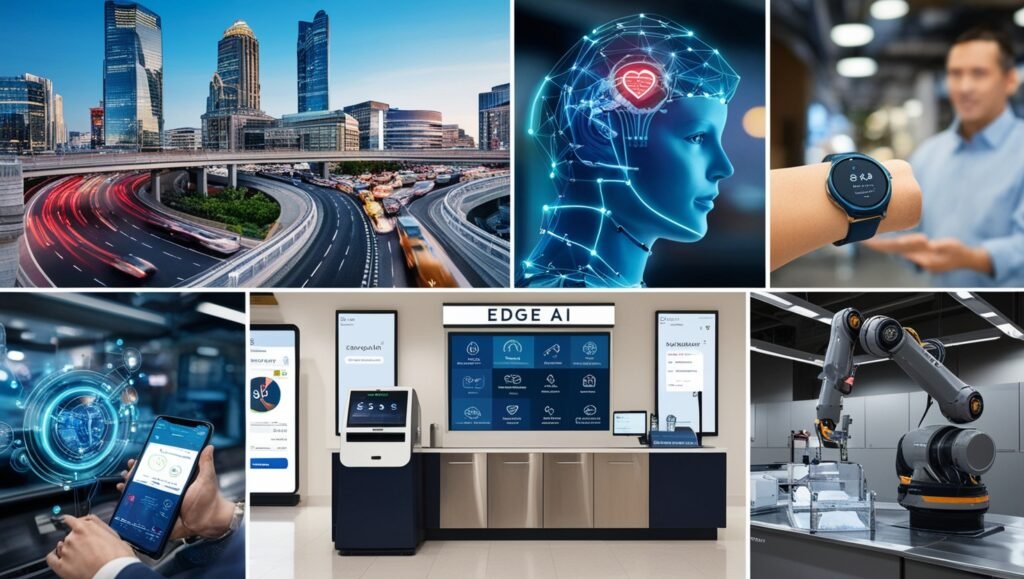
Healthcare
- Remote Health Monitoring: Wearables and IoT devices can monitor patients’ health metrics, using AI to detect anomalies or predict health events without sending data to a cloud.
- Telemedicine: Edge AI can support real-time diagnostics and consultations by processing medical imaging or patient data on the spot, enhancing the quality of remote healthcare.
- Drug Discovery: Lab equipment with edge AI can analyze experimental data on-site to speed up the drug development process.
Automotive and Transportation
- Autonomous Driving: Vehicles use edge AI for real-time decision-making, processing sensor data to navigate, avoid obstacles, and respond to traffic conditions.
- Fleet Management: AI at the edge can optimize routes, predict maintenance needs, and monitor driver behavior for logistics companies.
- Traffic Flow Optimization: Smart traffic systems use edge AI to adjust signal timings based on real-time traffic conditions.
Agriculture
- Precision Agriculture: Drones or sensors use edge AI to analyze soil and crop health, providing farmers with immediate insights for better resource management.
- Livestock Management: Wearable tech on animals can analyze behavior and health, alerting farmers to intervene when necessary.
Energy Management
- Smart Grids: Edge AI helps in real-time energy distribution management, fault detection, and integration of renewable energy sources.
- Energy Consumption: Smart meters with AI can predict usage patterns and suggest energy-saving measures directly to consumers.
Manufacturing
- Predictive Maintenance: Machines equipped with sensors use edge AI to predict when maintenance is needed,
Challenges of Edge AI
While Edge AI offers numerous benefits, it also faces various type of challenges such as limited computational resources akin to cellular constraints, energy efficiency struggles similar to metabolic processes, the complexity of model updates mirroring genetic evolution, and security vulnerabilities comparable to immune system weaknesses
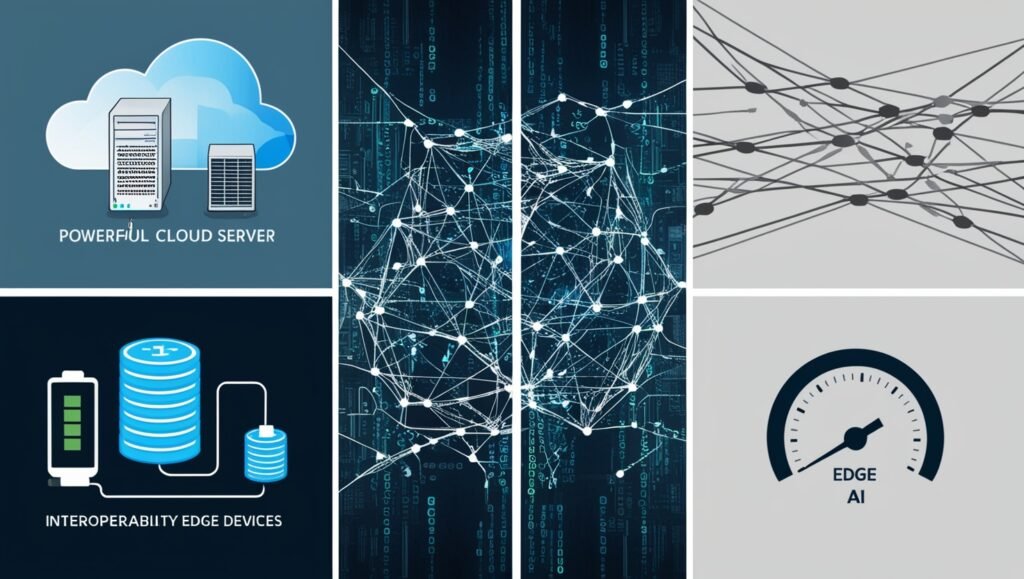
Resource Limitations:
Edge devices typically have less processing power, memory, and storage compared to cloud servers. This necessitates the development of extremely efficient AI models that can perform with these constraints while still delivering accurate results.
Energy Consumption:
Although Edge AI can lead to energy savings by reducing data transmission, the actual processing on the device can consume considerable power, especially for continuous operations or complex AI tasks. Balancing performance with energy efficiency remains a challenge.
Model Management and Updates:
Deploying and updating AI models on a large scale across many edge devices can be logistically complex. Ensuring all devices have the latest model without overwhelming network bandwidth or device storage is a significant issue.
Privacy vs. Utility:
While Edge AI enhances privacy by processing data locally, there’s a trade-off with the utility of data. For instance, the inability to aggregate data from multiple sources might limit the effectiveness of some AI algorithms that benefit from larger datasets.
Security Risks:
Edge devices can be more vulnerable to attacks since each device becomes a potential security point. Protecting these devices from tampering, ensuring secure boot processes, and safeguarding AI model integrity are critical areas of concern.
Data Quality and Bias:
With data being processed at the edge, there’s less opportunity for centralized data cleaning or bias correction. Ensuring the data quality and mitigating biases in edge environments can be more challenging.
Interoperability:
As different manufacturers produce edge devices with varying hardware capabilities, ensuring that AI models can run universally or be tailored to different hardware without significant customization is complex.
Scalability:
Scaling Edge AI solutions across thousands or millions of devices, each with potentially different environments and use cases, requires sophisticated management systems that can handle diversity and heterogeneity.
Regulatory Compliance:
With AI decisions being made at the edge, ensuring compliance with regulations like GDPR or HIPAA becomes more complex, especially regarding data handling, privacy, and consent.
Network Dependency for Some Functions:
While Edge AI reduces dependency on the network for processing, certain functionalities still require intermittent connectivity for model updates, synchronization, or data backup, which can be problematic in areas with poor connectivity.
Complexity in Development:
Developing AI for edge environments demands a different approach than cloud-based AI development. Developers need to consider the unique constraints of edge devices, leading to a steeper learning curve and potentially higher development costs.
The Future of Edge AI
Edge AI is poised to become an integral part of our technology ecosystem. As hardware and connectivity continue to improve, the adoption of Edge AI will expand across sectors. The integration of 5G and AI-driven platforms will unlock new possibilities, from personalized healthcare to autonomous systems.
Startups and tech giants are leading the charge, developing innovative solutions to bring AI processing power to the edge. With its potential to revolutionize industries, Edge AI is not just a technological advancement but a gateway to a smarter, more efficient future.